TL;DR: VirusTotal APIv3 includes an endpoint to retrieve all the dynamic analysis reports for a given file. This article showcases programmatic retrieval of sandbox behaviour reports in order to produce indicators of compromise that you can use to power-up your network perimeter/endpoint defenses. We are also releasing a set of python scripts alongside this blog post to illustrate this use case.
We recently rolled out a
new Windows dynamic analysis system called VirusTotal Jujubox. This new sandbox represents a major revamp of VirusTotal’s in-house behaviour analysis capabilities as well as a key addition to the
multi-sandbox project, which already aggregates behaviour reports from more than 10 partners and the most popular operating systems.
Behaviour reports are often perceived as a mechanism to understand what an individual sample does when executed, a quick overview before diving into disassembly and debugging. However, when you have a massive dynamic analysis setup processing hundreds of thousands of files per day, the microscopic dissection capability is far from being the most attractive use case.
When you generate
reports at scale, and more importantly, when you index them in an elasticsearch index and expose it via
API, the generated data can be used for advanced hunting, especially when this data can be combined with other static, binary and in-the-wild properties.
The basic workflow would be as follows:
- Periodically identify new malware variants pertaining to a family that you are tracking making use of the VT Intelligence search API. Use family variant commonalities (for instance a section name, the compilation timestamp or a document’s author metadata property) to retrieve a stream of malware.
- Focus on recent matches since the previous execution (query: fs:2019-11-01+).
- For each match, retrieve the generated behaviour reports for the pertinent file. You can also focus specifically on network communications with the contacted_ips, contacted_domains and contacted_urls relationships.
- For each automatically extracted network observable, check popularity ranks in order to filter out noise and FPs.
- All the newly yielded network artefacts (CnCs) can then be fed into SIEMs or transformed into IDS rules to power up network perimeter defenses.
Let’s illustrate this with a particular example.
Bankbot is an Android banking trojan, it allows the attacker to perform:
- SMS hijacking.
- GPS tracking.
- New permission requests.
- Overlay attacks to mask legit bank apps with forms to intercept credentials. Sometimes based on a remote set of HTML templates.
The trojan was released in an underground forum and the post included the source code for the client-side and server-side components, including the database setup to collect stolen information.
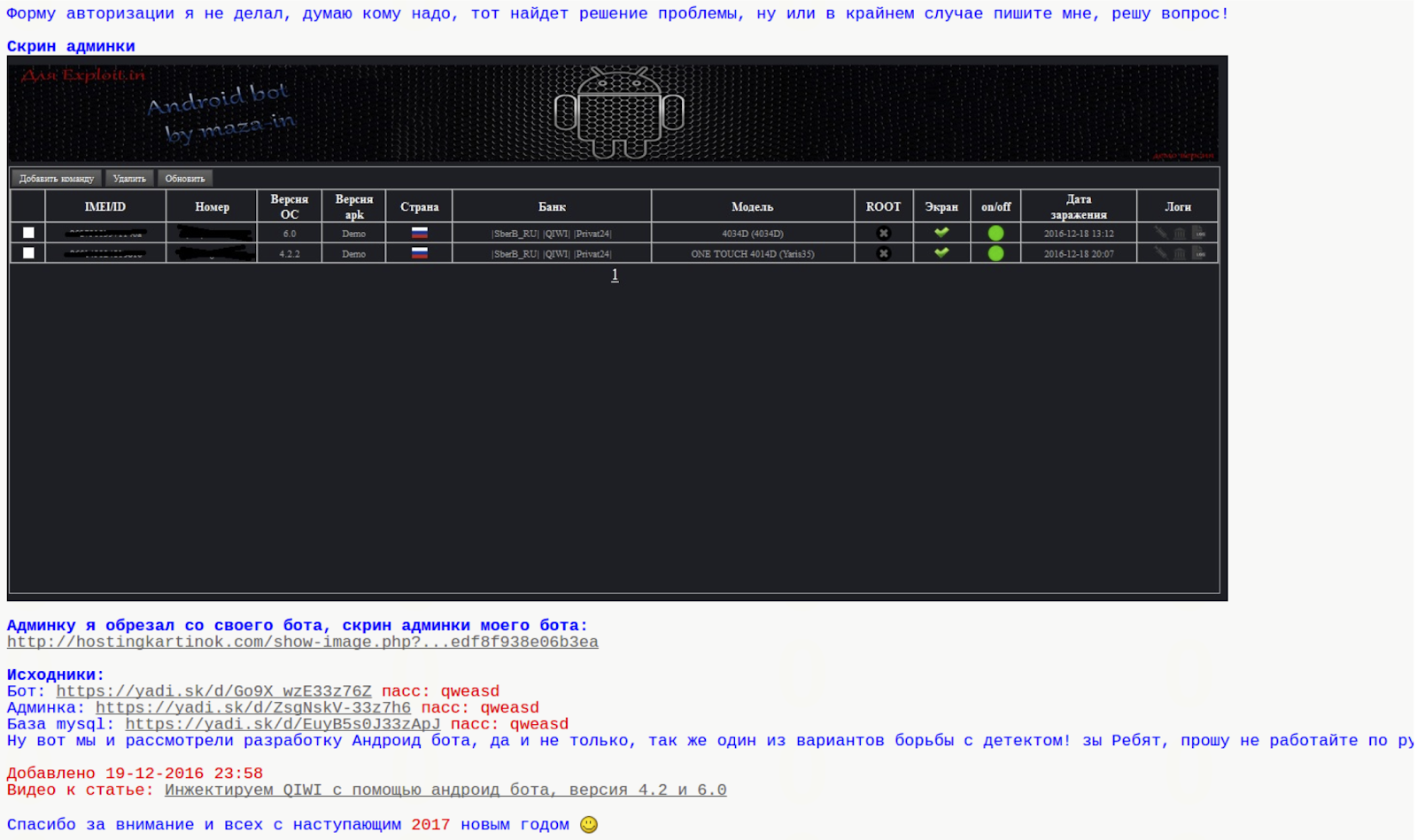
Initially, the trojan included a hardcoded list of target bank applications that it would overlay in order to intercept banking credentials:
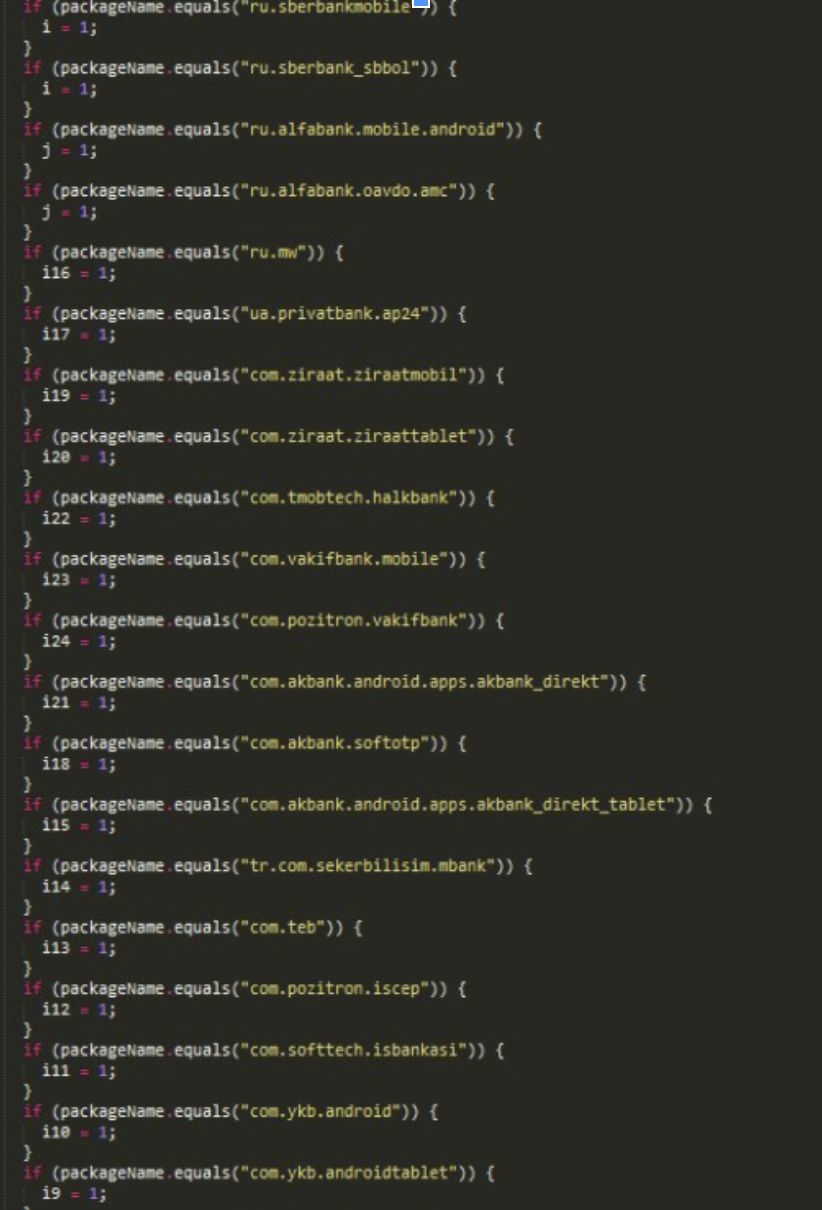
Since the source code of the trojan was also published in the underground forum, other crooks soon modified it to accept a remote list of financial entities to attack. This makes target identification more complex, static analysis is not enough to identify the targeted banks and subsequent date-tied CnC infrastructure.

While identifying targeted financial institutions might be a more complex task, discovering new variants of the same family and automatically identifying new network infrastructure tied to it becomes easier. Why is this? A server-side remote target list leads to a common network infrastructure pattern that can be used to track the malware family.
This is an example of a Bankbot sample:
https://www.virustotal.com/gui/file/5fdbe1e83ec9c43929cc348681cb6afde12afee637feaf444a4983c317b18423/detectionVT Enterprise allows similarity searches and other attribute searches to find additional variants of the same malware family. In this particular case, the Android package name under the
details tab seems interesting, clicking on it will launch a
VT Intelligence search for other Android APKs that share that very same package name:
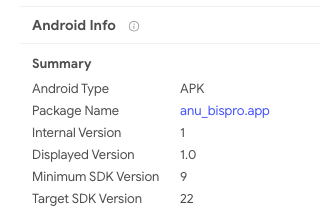
The matches do indeed seem to belong to the same family:
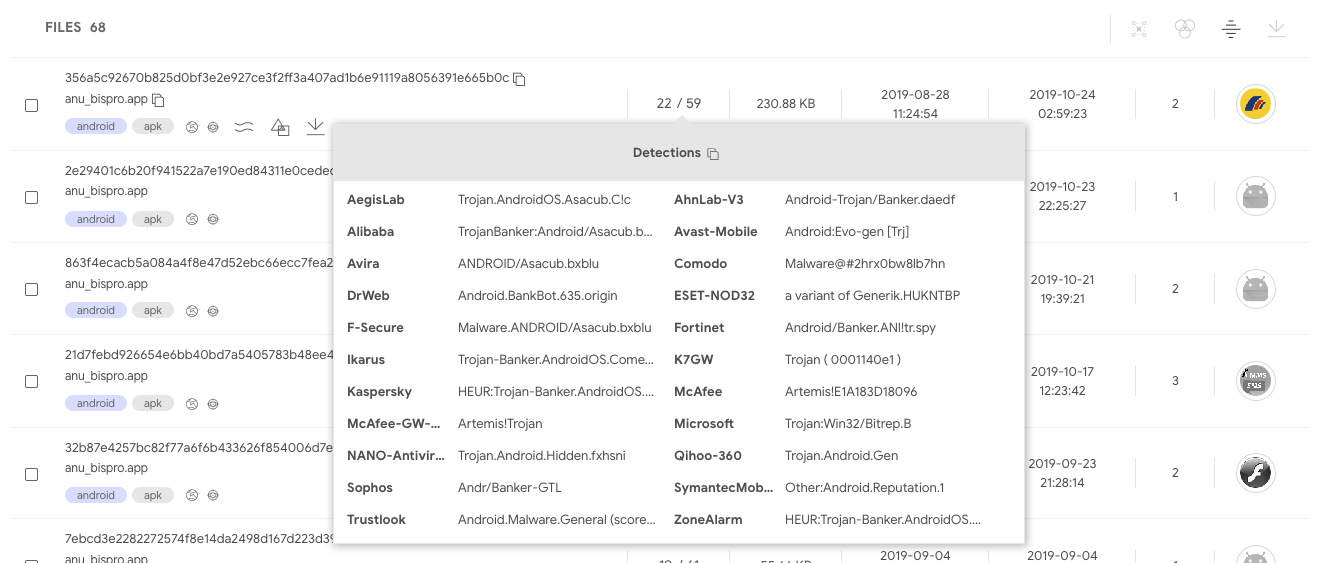
When opening these samples and looking at their
behaviour reports, certain commonalities are easily noticed:
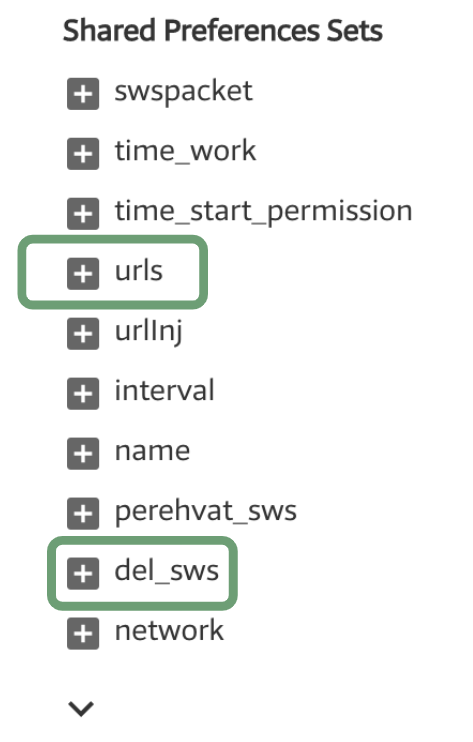

Static/behaviour/code commonalities are very frequent since attackers usually reuse code across different campaigns. Sometimes the commonalities are a result of recompiling the same code to communicate with a different network infrastructure. Other times, commonalities are present because the attack binaries are generated with some kind of builder or kit for dummies. Similarly, CnC infrastructure often exhibits commonalities in terms of the same path structure or query parameters, it is the result of attackers reusing the same CnC panel through a server-side kit that they deploy without changing file names or path structure.
These patterns, in conjunction with VT’s massive dynamic analysis setup and indexing, make it easy to automatically discover new malicious network infrastructure and automatically generate indicators of compromise.
The behaviour reports for the identified cluster of samples shows that the CnC panel uses the subpaths tuk_tuk.php or checkPanel.php.
Let’s use this common pattern to periodically check VirusTotal for new variants of this malware family, and by doing so, let’s identify new network infrastructure tied to this attack, live, as samples are uploaded to VirusTotal.
Using the
APIv3 Intelligence search endpoint, it’s possible to search for any Android APK whose network recordings contain the substring tuk_tuk.php:
https://developers.virustotal.com/v3.0/reference#intelligence-searchtype:apk behaviour_network:"tuk_tuk.php"Multiple properties, such as dynamic/static analysis and metadata, can be combined to make a more refined search:
type:apk behaviour_network:"tuk_tuk.php" behaviour:"del_sws" androguard:"android.permission.ACCESS_FINE_LOCATION"The API can sort matches according to first seen descending, meaning that by executing this search periodically and focusing on the latest results, it’s possible to discover new malicious network infrastructure tied to this particular family.
At the time of writing, this search yielded the following results:
5fdbe1e83ec9c43929cc348681cb6afde12afee637feaf444a4983c317b18423elis[.]ru
92.53.97[.]75
hxxp://elis.ru/private/tuk_tuk.php
52998a07d22b0aa267505635898219ef6104dc6cd255bea69c7ab701285666faxzcxzfs.kl.com[.]ua
5.79.66[.]145
hxxp://xzcxzfs.kl.com[.]ua/private/tuk_tuk.php
7c06552f59b594ef0d650204423e97c8ab8f07588f1215ec2a469dc9cb7f5670u36084.test93w[.]ru
hxxp://u36084.test93w[.]ru/private/tuk_tuk.php
56b220e610d17987b4f96afa79e23c3c9cab16592384ed883e9ac8240907b53bu36206.test93w[.]ru
185.31.163[.]148
hxxp://u36206.test93w[.]ru/private/tuk_tuk.php
The
Intelligence search API endpoint will return a list of file objects matching a search criteria. Each of these file objects can have one or more multi-sandbox reports. These behaviour reports can be retrieved making use of the pertinent relationship (behaviours) for each of the files:
https://developers.virustotal.com/v3.0/reference#files-relationshipsIt’s also possible to filter the network communication relationships fields, instead of asking for the whole report (contacted_urls, contacted_ips, contacted_domains):
https://www.virustotal.com/api/v3/intelligence/search?query=type:apk behaviour_network:”tuk_tuk.php”&relationships=contacted_urls,contacted_domains,contacted_ipsOnce the pertinent network infrastructure is parsed, it’s possible to either rely on the objects returned by the network-related relationships (contacted_urls, contacted_ips, contacted_domains) or make a subsequent automated call to the
domain /
IP address /
URL API endpoint in order to retrieve further details about the given network observable. The aim of this subsequent stage is to filter out potential false positives. For instance, among the details returned for a domain lookup, there are different popularity rank lists that can be useful to filter out TOP domains.
You can easily test this workflow with a
little script released along with this blog post. This script makes use of
our official APIv3 python library, it can serve as your starting point to build more complex pipelines:
https://github.com/VirusTotal/vt-py/blob/master/examples/intelligence_search_to_network_infrastructure.py
python3 intelligence_search_to_network_infrastructure.py --apikey=<YOUR_API_KEY> --query=’type:apk behaviour_network:"tuk_tuk.php"’
=== Results: ===
DOMAIN: u363571.test93w.ru
IP_ADDRESS: 185.31.163.148
URL: http://u363571.test93w.ru/private/tuk_tuk.php
DOMAIN: bot.mymaster-rem.ru
URL: http://bot.mymaster-rem.ru/private/tuk_tuk.php
DOMAIN: lensfor.xyz
URL: https://lensfor.xyz/private/tuk_tuk.php
IP_ADDRESS: 38.21.243.204
URL: http://38.21.243.204/anib/private/tuk_tuk.php
DOMAIN: f0316480.xsph.ru
IP_ADDRESS: 141.8.192.151
URL: http://f0316480.xsph.ru/private/tuk_tuk.php
DOMAIN: u36255.test93w.ru
DOMAIN: mtalk4.google.com
IP_ADDRESS: 185.31.163.148
URL: http://u36255.test93w.ru/private/tuk_tuk.php
DOMAIN: u36206.test93w.ru
IP_ADDRESS: 185.31.163.148
URL: http://u36206.test93w.ru/private/tuk_tuk.php
DOMAIN: yumishop.co.uk
URL: http://yumishop.co.uk/private/inj_lst.php
URL: http://yumishop.co.uk/private/tuk_tuk.php
DOMAIN: u36317.test93w.ru
IP_ADDRESS: 185.31.163.148
URL: http://u36317.test93w.ru/private/tuk_tuk.php
DOMAIN: u36317.test93w.ru
IP_ADDRESS: 185.31.163.148
URL: http://u36317.test93w.ru/private/tuk_tuk.phpNote that this workflow is exclusively based on behavioural observations and works independently of the detection ratio of files, by pipelining
VT Intelligence searches and sandbox report lookups, it is possible to generate indicators of compromise even if the related sample is undetected. The identified domains can be automatically checked against SIEM logs or can be automatically transformed into IDS rules, serving as an additional layer in your onion-like security strategy.
This blog post focuses on combining
VT Intelligence searches with behaviour lookups, the same can be done with YARA rule matches.
VT Hunting Livehunt matches can programmatically retrieved using
APIv3, for each match the pertinent behaviour reports can be retrieved and CnC network infrastructure can be automatically extracted. Similarly, other properties that can be used as IoCs, such as mutexes, registry keys, embedded domains, file names, cmd parameters and the like can be automatically yielded. The following two script showcase this other
VT Hunting workflow:
https://github.com/VirusTotal/vt-py/blob/master/examples/hunting_notifications_to_network_infrastructure.pyhttps://github.com/VirusTotal/vt-py/blob/master/examples/retrohunt_to_network_infrastructure.pyIf you are rather a golang fan, feel free to check out our official VirusTotal golang library:
https://github.com/VirusTotal/vt-go
APIv3 was a major component of our
2019 roadmap, soon we will be officially releasing it and announcing a generous deprecation timeline for APIv2, stay tuned!